How to transfer a Python graph to a Neo4j graph
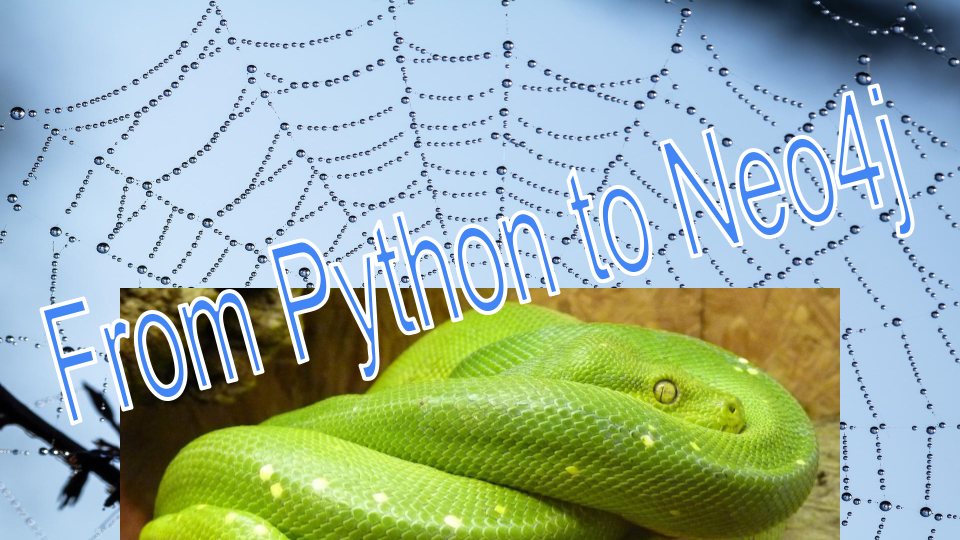
- Data concerning publications found on the data.world website
- Data concerning the Olympic winter sport competitions found in the data.world website
- Music data found in kaggle website
The objective here is to generate the csv files which will represent the corpus (that will be treated in Corpus_pre_processing.ipynb) and the graphs that I will imported into neo4j.
import pandas as pd
from pandarallel import pandarallel
pandarallel.initialize()
New pandarallel memory created - Size: 2000 MB
Pandarallel will run on 8 workers
Data about publications:
These data come from roswell park cancer institute that I found in the data.world website here
articles = pd.read_csv("data/publications-from-roswell-park-cancer-institute-beginning-2006-1.csv")
articles.head()
articles.info()
<class ‘pandas.core.frame.DataFrame’>
RangeIndex: 10147 entries, 0 to 10146
Data columns (total 11 columns):
Year Published 10147 non-null int64
Publication Type 10147 non-null object
Journal Name 10116 non-null object
Publication Title 10147 non-null object
Author List 10145 non-null object
Journal Volume 9867 non-null float64
Journal Issue Number 9227 non-null object
Journal Page Range 10103 non-null object
ISSN 9850 non-null object
Peer Reviewed 10147 non-null object
Impact Factor 7753 non-null object
dtypes: float64(1), int64(1), object(9)
memory usage: 872.1+ KB
Check if there are any problems in the data!
articles["Peer Reviewed"].value_counts()
TRUE 7118
1 2705
FALSE 278
0 46
Name: Peer Reviewed, dtype: int64
articles.columns
Index([‘Year Published’, ‘Publication Type’, ‘Journal Name’,
‘Publication Title’, ‘Author List’, ‘Journal Volume’,
‘Journal Issue Number’, ‘Journal Page Range’, ‘ISSN’, ‘Peer Reviewed’,
‘Impact Factor’],
dtype=‘object’)
I will choose the columns that will be used to create the ontology.
articles = articles[['Journal Name', 'Publication Title', 'Author List', 'Peer Reviewed']]
articles.dropna(inplace=True)
articles.reset_index(drop=True, inplace = True)
articles.head()
Put peer reviewed or not peer reviewed in the column Peer Reviewed
def peer(x):
if x == '1' or x == 'TRUE' or x == 1:
return "peer reviewed"
elif x == '0' or x == 'FALSE' or x == 0:
return "not peer reviewed"
articles['Peer Reviewed'] = articles['Peer Reviewed'].parallel_apply(peer)
articles.head()
Add a column Topic
The topic will be an attribute of the authors and also of the articles in the ontology.
def preprocessing(text, result = 'str'):
from string import punctuation
from nltk.tokenize import word_tokenize
from nltk.corpus import stopwords
import slugify
remove_terms = punctuation + '0123456789'
words = word_tokenize(text)
tokens = [w for w in words if w.lower() not in remove_terms]
stopw = stopwords.words('english')
tokens = [token for token in tokens if token not in stopw]
# remove words less than one letters
tokens = [word for word in tokens if len(word)>2]
# remove remaining tokens that are not alphabetic
tokens = [word for word in tokens if word.isalpha()]
tokens = [slugify.slugify(word) for word in tokens]
if result == 'str':
tokens = ' '.join(tokens)
return tokens
articles['title_topic'] = articles['Publication Title'].parallel_apply(preprocessing)
articles.head()
all_unique_words_in_titles = set()
for i in articles.index:
all_unique_words_in_titles.add(articles.iloc[i]["title_topic"])
all_unique_words_in_titles = preprocessing(' '.join(list(all_unique_words_in_titles)), list)
print("Number of unique words in titles:", len(all_unique_words_in_titles))
Number of unique words in titles: 94650
To extract topics from a text, normally we use the techniques of Named Entity Recognition or Latent Dirichlet Allocation using libraries such as NLTK or spaCy or transfer learning based on pre-trained models like Bert… But due to lack of time, I will use the FastText library and using the similarity, I will create my Topic column
from gensim.models.fasttext import FastText
from nltk import WordPunctTokenizer
wpt = WordPunctTokenizer()
tokenized_corpus = [wpt.tokenize(doc) for doc in all_unique_words_in_titles]
feature_size = 500 # Word embedding vector dimensionality
window_context = 5 # Context window size
min_word_count = 2 #2 # Minimum word count
sample = 1e-3 # Downsample setting for frequent words
fasttext_model_sg = FastText(tokenized_corpus,
size=feature_size,
window=window_context,
min_count=min_word_count,
sample=sample,
sg=1, # sg decides whether to use the skip-gram model (1) or CBOW (0)
iter=100)
def title_topic(title):
if len(title.split(" ")) > 1:
return fasttext_model_sg.wv.most_similar(title)[0][0]
if len(title.split(" ")) == 1:
return title
from collections import defaultdict
title_dict = defaultdict(list)
for i in articles.index:
try:
title_dict["title_topic"].append(title_topic(articles.iloc[i]["title_topic"]))
except:
title_dict["title_topic"].append("")
articles["Topic"] = pd.Series(title_dict)["title_topic"]
articles = articles[articles["title_topic"] != '']
articles = articles[articles["Topic"] != '']
articles = articles.reset_index(drop=True)
articles.drop(columns=['title_topic'], inplace=True)
articles.head()
articles.drop_duplicates(inplace=True)
Normally I have to create my ‘knwoldege Graph’ from the corpus that I will generate at the end of this jupyter notebook, but for reasons that I will explain in a second jupyter notebook Corpus_pre_processing.ipynb, I will generate csv files that will be used to create the publications part of my graph on neo4j.
Warning: On my laptop the Cypher queries crash if I take all the data. To give you a live demonstration without any problem, I will only take the first 100 rows of the DataFrame articles.
Nodes that represent the authors and their attributes
The attribues of an author are:
- name
- topic
- article title
author = articles[['Author List', 'Topic', 'Publication Title']].head(100)
author.rename(columns={'Author List': "name", 'Topic': "topic", 'Publication Title': 'article title'}, inplace=True)
def string_to_list(x):
return x.split(';')
author['name'] = author['name'].parallel_apply(string_to_list)
author.head()
author.info()
<class ‘pandas.core.frame.DataFrame’>
RangeIndex: 100 entries, 0 to 99
Data columns (total 3 columns):
name 100 non-null object
topic 100 non-null object
article title 100 non-null object
dtypes: object(3)
memory usage: 2.5+ KB
import numpy as np
Convert author to long form
author = pd.DataFrame({
col:np.repeat(author[col].values, author['name'].str.len())
for col in author.columns.drop('name')}
).assign(**{'name':np.concatenate(author['name'].values)})[author.columns]
author.head()
author.info()
<class ‘pandas.core.frame.DataFrame’>
RangeIndex: 1240 entries, 0 to 1239
Data columns (total 3 columns):
name 1240 non-null object
topic 1240 non-null object
article title 1240 non-null object
dtypes: object(3)
memory usage: 29.2+ KB
author = author[['name', 'topic']]
author.drop_duplicates(inplace=True)
/home/arij/anaconda3/lib/python3.6/site-packages/ipykernel_launcher.py:1: SettingWithCopyWarning:
A value is trying to be set on a copy of a slice from a DataFrame
See the caveats in the documentation: http://pandas.pydata.org/pandas-docs/stable/user_guide/indexing.html#returning-a-view-versus-a-copy
“”"Entry point for launching an IPython kernel.
author.info()
<class ‘pandas.core.frame.DataFrame’>
Int64Index: 1234 entries, 0 to 1239
Data columns (total 2 columns):
name 1234 non-null object
topic 1234 non-null object
dtypes: object(2)
memory usage: 28.9+ KB
Nodes that represent the articles and their attributes
The attribues of an article are:
- article title
- topic
- published in
- authors
article = articles[['Publication Title', 'Topic', 'Journal Name', 'Author List']].head(100)
article.rename(columns={'Publication Title': 'article title', 'Topic': "topic", 'Journal Name': 'published in', 'Author List': 'authors'}, inplace=True)
article.head()
article.info()
<class ‘pandas.core.frame.DataFrame’>
Int64Index: 100 entries, 0 to 99
Data columns (total 4 columns):
article title 100 non-null object
topic 100 non-null object
published in 100 non-null object
authors 100 non-null object
dtypes: object(4)
memory usage: 3.9+ KB
article.drop_duplicates(inplace=True)
Nodes that represent the journals and their attributes
The attribues of a journal are:
- name
- evaluation
journal = articles[['Journal Name', 'Peer Reviewed']].head(100)
journal.rename(columns={'Journal Name': 'name', 'Peer Reviewed': 'evaluation'}, inplace=True)
journal.head()
journal.info()
<class ‘pandas.core.frame.DataFrame’>
RangeIndex: 100 entries, 0 to 99
Data columns (total 2 columns):
name 100 non-null object
evaluation 100 non-null object
dtypes: object(2)
memory usage: 1.7+ KB
journal.drop_duplicates(inplace=True)
journal.info()
<class ‘pandas.core.frame.DataFrame’>
Int64Index: 77 entries, 0 to 98
Data columns (total 2 columns):
name 77 non-null object
evaluation 77 non-null object
dtypes: object(2)
memory usage: 1.8+ KB
journal.to_csv("journal.csv", encoding='utf-8', index=False)
article.to_csv("article.csv", encoding='utf-8', index=False)
author.to_csv("author.csv", encoding='utf-8', index=False)
Data about music:
These data come from kaggle website here
music = pd.read_csv("data/top50.csv", engine='python')
music.head()
music.info()
<class ‘pandas.core.frame.DataFrame’>
RangeIndex: 50 entries, 0 to 49
Data columns (total 14 columns):
Unnamed: 0 50 non-null int64
Track.Name 50 non-null object
Artist.Name 50 non-null object
Genre 50 non-null object
Beats.Per.Minute 50 non-null int64
Energy 50 non-null int64
Danceability 50 non-null int64
Loudness…dB… 50 non-null int64
Liveness 50 non-null int64
Valence. 50 non-null int64
Length. 50 non-null int64
Acousticness… 50 non-null int64
Speechiness. 50 non-null int64
Popularity 50 non-null int64
dtypes: int64(11), object(3)
memory usage: 5.6+ KB
Warning: This data only contains 50 rows! Once in the corpus will make it unbalanced. This means that to clustering on the corpus for sharding purposes, you have to increase the data or at least add weights in the training set (but with 50 rows, adding weights in the training set will not be enough, it is necessary to add music data).
music["Danceability"].value_counts()
music["Liveness"].value_counts()
music["Valence."].value_counts()
music.columns
music = music[['Track.Name', 'Artist.Name', 'Genre', 'Liveness', 'Valence.']]
music.drop_duplicates(inplace=True)
music.dropna(inplace=True)
music.reset_index(drop=True, inplace = True)
music.head()
I do some suitable transformations.
def mood(x):
if x <= 1:
return "negative"
else:
return "positive"
def live(x):
if x <= 1:
return "no"
else:
return "yes"
music['Valence.'] = music['Valence.'].parallel_apply(mood)
music['Liveness'] = music['Liveness'].parallel_apply(live)
music.head()
Nodes that represent the artist and their attributes
The attribues of an artist are:
- name
- track
- genre
artist = music[['Artist.Name', 'Track.Name', 'Genre']]
artist.rename(columns={'Artist.Name': 'name', 'Track.Name': 'track', 'Genre': 'genre'}, inplace=True)
artist.head()
Nodes that represent the song and their attributes
The attribues of a song are:
- track
- genre
- mood
song = music[['Track.Name', 'Genre', 'Valence.']]
song.rename(columns={'Track.Name': 'track', 'Genre': 'genre', 'Valence.': 'mood'}, inplace=True)
song.head()
artist.to_csv("artist.csv", encoding='utf-8', index=False)
song.to_csv("song.csv", encoding='utf-8', index=False)
Data about athletes:
These data come from athletes in winter olympics that I found in the data.world website here
olympics = pd.read_excel("data/Winer Olympic Medals.xlsx")
olympics.head()
olympics.info()
olympics.columns
Index([‘Year’, ‘Sport’, ‘Event’, ‘Country’, ‘Gender’, ‘Medal Rank’, ‘Medal’,
‘Name of Athlete or Team’, ‘Age of Athlete’],
dtype=‘object’)
olympics = olympics[['Year', 'Sport', 'Country', 'Gender', 'Medal', 'Name of Athlete or Team', 'Age of Athlete']]
olympics.drop_duplicates(inplace=True)
olympics.dropna(inplace=True)
olympics.info()
olympics.reset_index(drop=True, inplace = True)
olympics.head()
Warning: On my laptop the Cypher queries crash if I take all the data. To give you a live demonstration without any problem, I will only take the first 50 rows of the DataFrame olympics.
Nodes that represent the athlete and their attributes
The attribues of an athlete are:
- name
- gender
- age
- sport
- country
- medal
- year
athlete = olympics[['Name of Athlete or Team', 'Gender', 'Age of Athlete', 'Sport', 'Country', 'Medal', 'Year']].head(50)
athlete.rename(columns={'Name of Athlete or Team': 'name', 'Gender': 'gender', 'Age of Athlete': 'age', 'Sport': 'sport','Country': 'country', 'Medal': 'medal', 'Year': 'year'}, inplace=True)
athlete.drop_duplicates(inplace=True)
athlete.reset_index(drop=True, inplace = True)
athlete.head()
athlete.info()
Nodes that represent the sport and their attributes
The attribues of a sport are:
- sport
sport = olympics[['Sport']].head(50)
sport.rename(columns={'Sport': 'sport'}, inplace=True)
sport.drop_duplicates(inplace=True)
sport.dropna(inplace=True)
sport.reset_index(drop=True, inplace = True)
sport.info()
sport.head()
Nodes that represent the medal and their attributes
The attribues of a medal are:
- medal
medal = olympics[['Medal']].head(50)
medal.rename(columns={'Medal': 'medal'}, inplace=True)
medal.drop_duplicates(inplace=True)
medal.dropna(inplace=True)
medal.reset_index(drop=True, inplace = True)
medal.info()
<class ‘pandas.core.frame.DataFrame’>
RangeIndex: 3 entries, 0 to 2
Data columns (total 1 columns):
medal 3 non-null object
dtypes: object(1)
memory usage: 152.0+ bytes
medal.head()
athlete.to_csv("athlete.csv", encoding='utf-8', index=False)
sport.to_csv("sport.csv", encoding='utf-8', index=False)
medal.to_csv("medal.csv", encoding='utf-8', index=False)